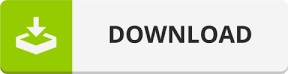
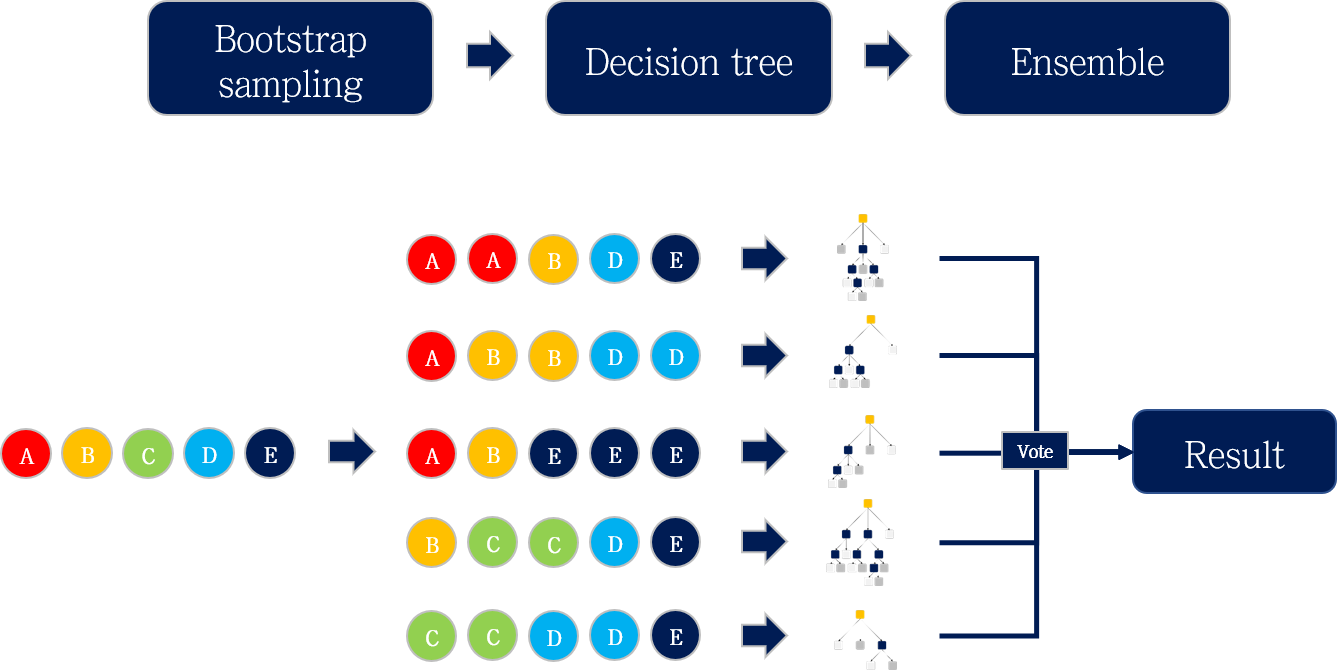
Xgboost random forest how to#
Here is an instance of how to calculate the weights of the leaf nodes in XGB-Ĭonsider a test data point, where age=10 and gender=female.To get the prediction for the data point, the tree is traversed from the top to bottom, performing a series of tests. Solution: The “leaf weight” can be said as the model’s predicted output associated with each leaf (exit) node. 4| What does the weight of XGB leaf nodes mean? How to calculate it? The training then proceeds iteratively, adding new trees with the capability to predict the residuals as well as errors of prior trees that are then coupled with the previous trees to make the final prediction.Ĭlick here to learn the step by step process of how XGB works. XGB minimises a regularised objective function that merges a convex loss function, which is based on the variation between the target outputs and the predicted outputs. Solution: When using gradient boosting for regression, where the weak learners are considered to be regression trees, each of the regression trees maps an input data point to one of its leaves that includes a continuous score. Unlike LightGBM, in XGB, one has to manually create dummy variable/ label encoding for categorical features before feeding them into the models.Like any other boosting method, XGB is sensitive to outliers.It provides various intuitive features, such as parallelisation, distributed computing, cache optimisation, and more.XGBoost has an in-built capability to handle missing values.XGB consists of a number of hyper-parameters that can be tuned - a primary advantage over gradient boosting machines.2| What are the advantages and disadvantages of XGBoost? This is the reason why XGBoost generally performs better than random forest. Meaning, each of the trees is grown using information from previously grown trees, unlike bagging, where multiple copies of original training data are created and fit separate decision tree on each. Random forest builds trees in parallel, while in boosting, trees are built sequentially. Also, random forest can be used as a base model for gradient boosting techniques.įurther, random forest is an improvement over bagging that helps in reducing the variance.
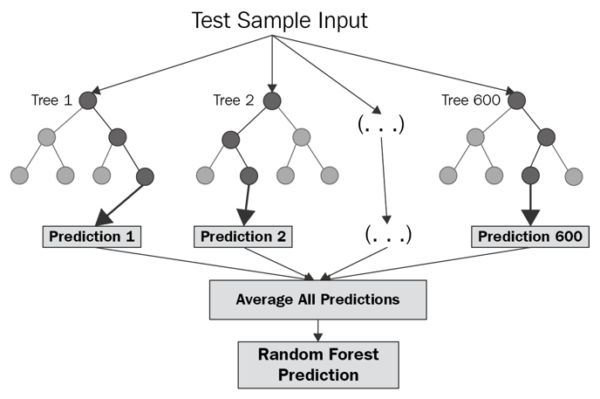
XGBoost can be used to train a standalone random forest. Random forests also use the same model representation and inference as gradient-boosted decision trees, but it is a different training algorithm. Solution: XGBoost is usually used to train gradient-boosted decision trees (GBDT) and other gradient boosted models. Here are the top ten interview questions on XGBoost that Data Scientists must know. It implements ML algorithms under the Gradient Boosting framework and helps in solving data science problems in a fast and accurate manner. The method is used for supervised learning problems and has been widely applied by data scientists to get optimised results for various machine learning challenges. XGBoost is an optimised distributed gradient boosting library, which is highly efficient, flexible and portable. Introduced a few years ago by Tianqi Chen and his team of researchers at the University of Washington, eXtreme Gradient Boosting or XGBoost is a popular and efficient gradient boosting method.
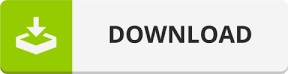